We advance research in mathematics: the science of numbers, shapes, probability and change.
The U.S. National Science Foundation is the leading supporter of fundamental mathematics research in the United States.
Whether it's unraveling the chaos of turbulence to better predict weather, accelerating the development of life-saving drugs, or improving the security and efficiency of the modern power grid, NSF research in mathematics keeps the U.S. at the leading edge of science and engineering.
What we support
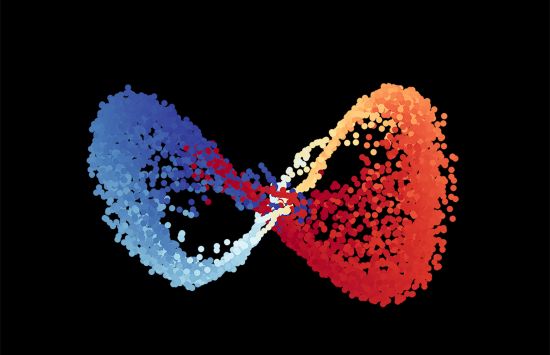
Innovation in mathematics and statistics
We support research on the properties of mathematical structures and how to use mathematics to solve problems in science, engineering and society at large.
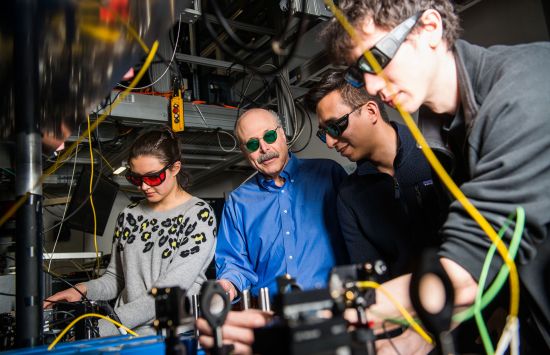
World-class research institutes
We support national mathematical research institutes that advance fundamental research and address problems in other disciplines.
Education and training
We support the next generation of mathematical scientists and statisticians through internships, fellowships, institutes and other programs.
Featured news
Resources